
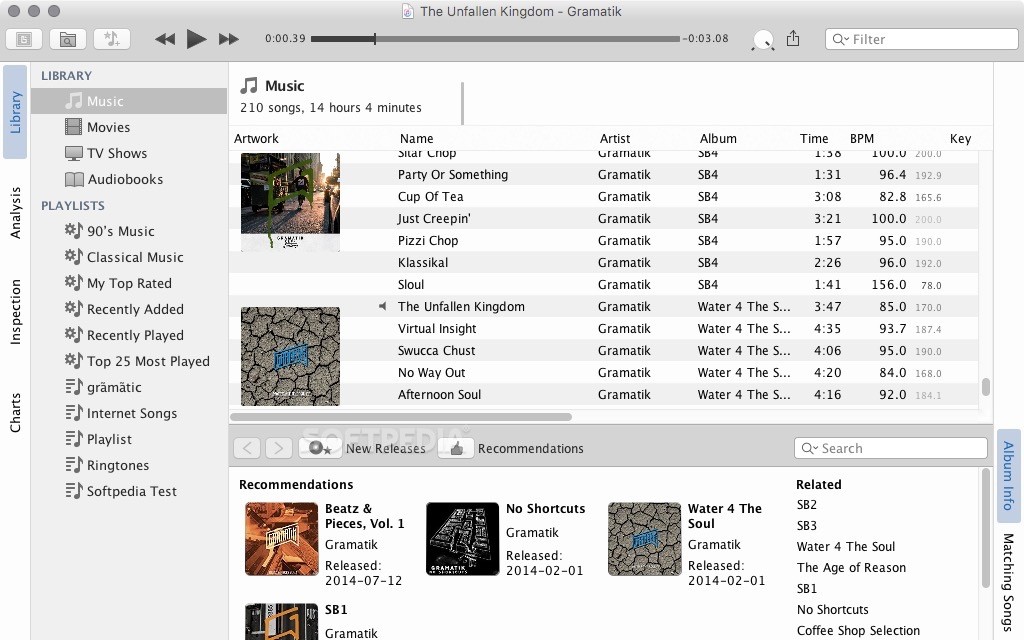
For training and evaluation the proposed data-driven approaches rely on curated datasets covering certain key and tempo ranges as well as genres. In particular, we investigate the effects of learning on different splits of cross-version datasets, i.e., datasets that contain multiple recordings of the same pieces. To improve our understanding of these systems, we systematically explore network architectures for both global and local estimation, with varying depths and filter shapes, as well as different ways of splitting datasets for training, validation, and testing. We find that the same kinds of networks can also be used for key estimation by changing the orientation of directional filters.
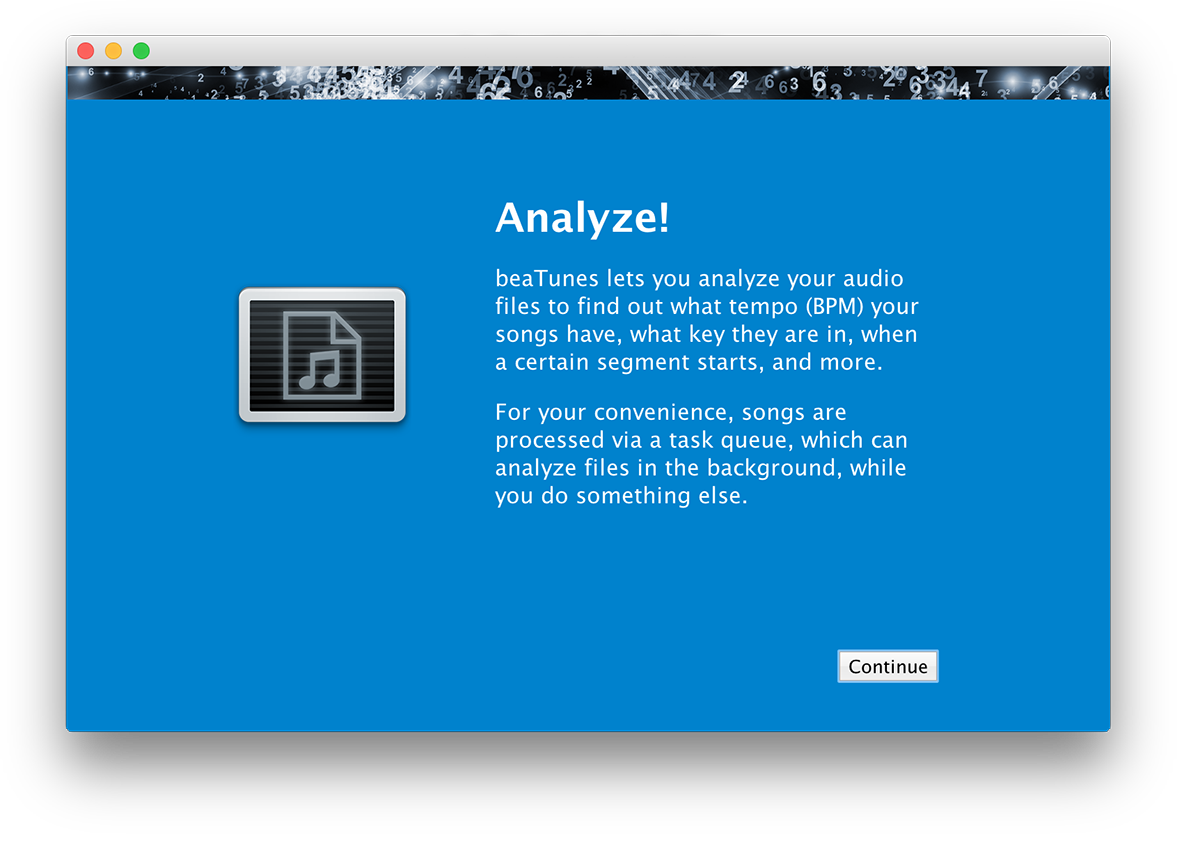
This allows us to take a purely data-driven approach using supervised machine learning (ML) with convolutional neural networks (CNN). We then re-formulate the signal-processing pipeline as a deep computational graph with trainable weights. We first propose novel methods using digital signal processing and traditional feature engineering. To improve tempo estimation, we focus mainly on shortcomings of existing approaches, particularly estimates on the wrong metrical level, known as octave errors. Both tasks are well established in MIR research. Key estimation labels music recordings with a chord name describing its tonal center, e.g., C major. Tempo estimation is often defined as determining the number of times a person would “tap” per time interval when listening to music. In this thesis, we propose, explore, and analyze novel data-driven approaches for the two MIR analysis tasks tempo and key estimation for music recordings. Creating such methods is a central part of the research area Music Information Retrieval (MIR). Efficient retrieval from such collections, which goes beyond simple text searches, requires automated music analysis methods. In recent years, we have witnessed the creation of large digital music collections, accessible, for example, via streaming services. To facilitate further research, all derived genre annotations are publicly available on our website. This both promises a more reliable ground truth and allows the evaluation of the newly generated and pre-existing datasets. We then combine multiple datasets using majority voting. These are most often used in MGR systems. Based on label co-occurrence rates, we derive taxonomies, which allow inference of top-level genres. In this paper we present a method for creating additional genre annotations for the MSD from databases, which contain multiple, crowd-sourced genre labels per song (Last.fm, beaTunes). Thus far, the quality of these annotations has not been evaluated. Therefore, multiple attempts have been made to add song-level genre annotations, which are required for supervised machine learning tasks. Another dataset, the Million Song Dataset (MSD), a collection of features and metadata for one million tracks, unfortunately does not contain readily accessible genre labels. Recently, the public dataset most often used for this purpose has been proven problematic, because of mislabeling, duplications, and its relatively small size.
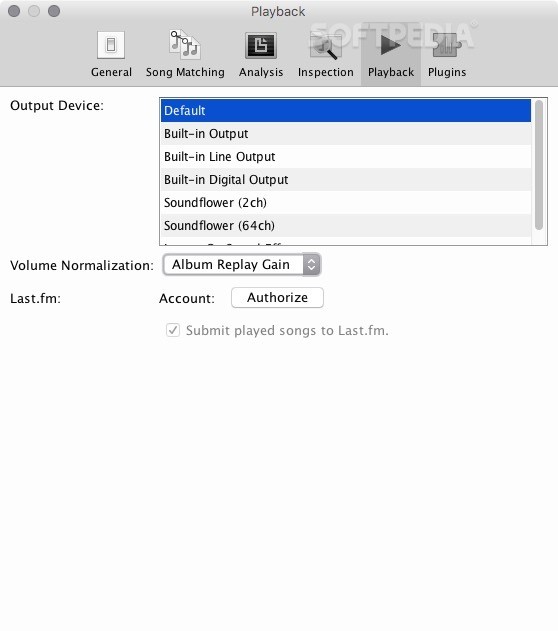
Any automatic music genre recognition (MGR) system must show its value in tests against a ground truth dataset.
